Non-small cell lung cancer (NSCLC) accounts for 85% of all lung cancers and can be divided into three histological subtypes with distinct presentation and prognoses: adenocarcinoma (ADC), squamous cell carcinoma (SCC), and large cell carcinoma (LCC).1 In addition to the histological differences, there is a remarkable degree of genetic variability within each subtype, emphasizing the importance of molecular biology and genotyping for NSCLC.
Machine Learning and Artificial Intelligence in NSCLC Patient Stratification
Artificial intelligence (AI) and machine learning (ML) methodologies have been helpful tools for stratifying NSCLC patients by predicting transcriptional mutations based on histological slides or discriminating subtypes through genomic expression levels. Traditional ML methodologies such as deep neural networks, require large datasets to distinguish ADC and SCC based on the molecular abnormalities associated with each.
Advantage of NetraAI for NSCLC Patient Stratification
To identify novel driving genes that distinguish ADC and SCC subtypes, a combination of ML tools was designed to learn from patient datasets to analyze gene expression data derived from NSCLC patients.2 ML with statistical modelling tailored for small datasets has shown promise in highlighting disease heterogeneity.3 Using NetraAI, which is designed for small datasets, we highlight a novel way of hypothesizing genetic subpopulations that may result in pathogenesis. The novelty of this approach is the ability to discover previously unknown subpopulations that are defined by several genes at a time that can shed light on the different mechanisms at play within these subtypes.
NetraAI and Hypothesis-Generation Uncovers Heterogeneity in NSCLC Subtypes
We used two publicly available NSCLC datasets containing 40 samples of ADC and 18 samples of SCC (GSE10245) and 14 samples of ADC and 32 samples of SCC (GSE18842) to obtain a total of 104 samples.4,5 Using a suite of ML techniques appropriate for small datasets, we had excellent signal for separating ADC and SCC. By using a smaller dataset, where the patients are unlikely to reflect the distribution of patients in the totality of reality, the ML methods can generate hypotheses about the population within the smaller dataset. In this way, the power of statistics is harnessed, where researchers can test the hypothesis and derive some measure of confidence. NetraAI empower this hypothesis testing paradigm.
Replicating Genetic Drivers Discriminating Between NSCLC Adenocarcinoma and Squamous Cell Carcinoma
This study highlights the genetic heterogeneity within NSCLC subtypes. Using these 2 datasets, a set of 10 genes that distinguish ADC (red) and SCC (blue) were identified (Figure 1). Within these two loops, SCC (Loop 1) was characterized by DSC3, VSNL1, SLC6A10P, IRF6, DST, CLCA2, DSG3, CGN, and PIGX. In contrast, ADC (Loop 2) was characterized by LPCAT1 overexpression.
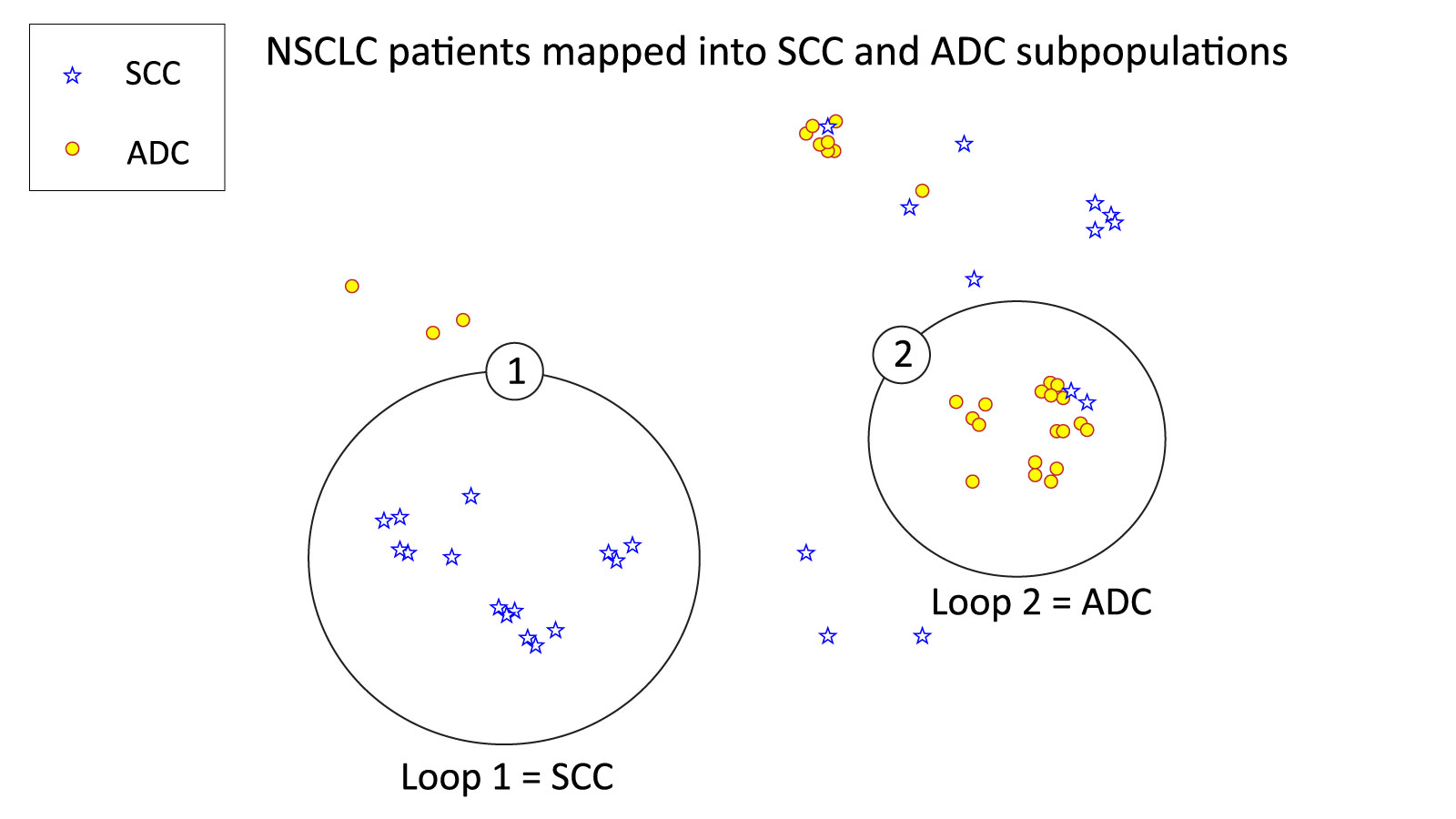
Figure 1. NetraAI stratification of NSCLC patients into SCC and ADC.
Of these 10 genes we identified to be genetic drivers distinguishing ADC and SCC, 9 have been previously reported to be differentially expressed in ADC or SCC, validating this ML approach (Table 1). These findings were aligned with previous reports on SCC genes being associated with the organization and assembly of cell and gap junctions, glutathione conjugation and the redox stress response, ECM organization and collagen-related proteins, interferon and cytokine signaling, and HLA downregulation and ADC genes associated with ECM organization proteins and complement, interferon and cytokine signaling, and collagen-related genes and proteins for ECM organization.6
Table 1. Genes discriminating between squamous cell carcinoma and adenocarcinoma
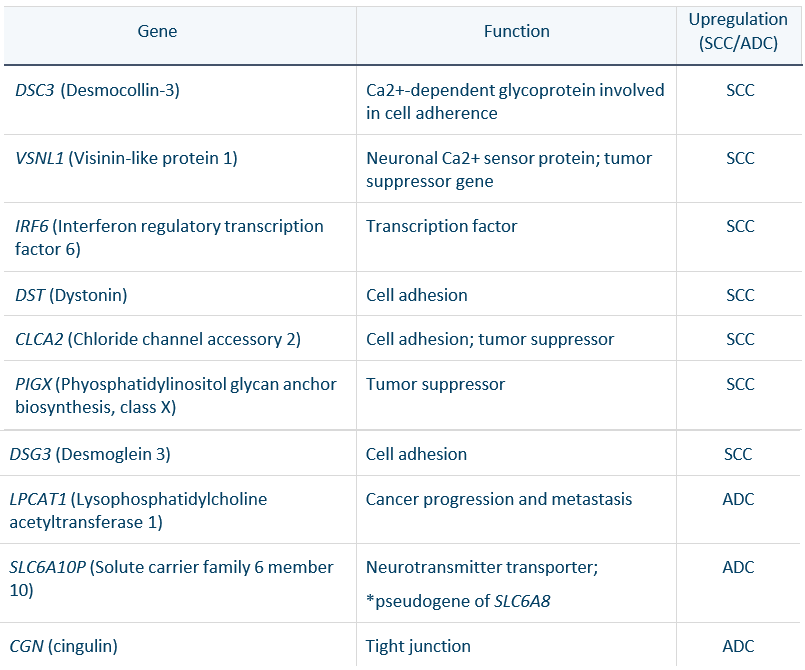
Uncovering Distinct Cellular Adhesion Molecules Associated with ADC and SCC
SCC has been reported to be characterized by upregulation of desmosome and gap junction genes, while ADC is reported to be characterized by the upregulation of tight junction genes.7 These reports suggest that NSCLC subtypes are associated with a distinct set of adhesion molecules. Using the 2 datasets, we found that SCC was associated with adhesion cell marker DSC3, and ADC was associated with tight junction marker CGN (Figure 2). Two probes were identified for each gene, and both probes corresponding to each gene were elevated. When looking at this expression profile across sex, elevated expression of DSC3 was associated with males, while elevated CGN was significantly associated with females. This unique finding highlights a potential role of sex-based differences in NSCLC.
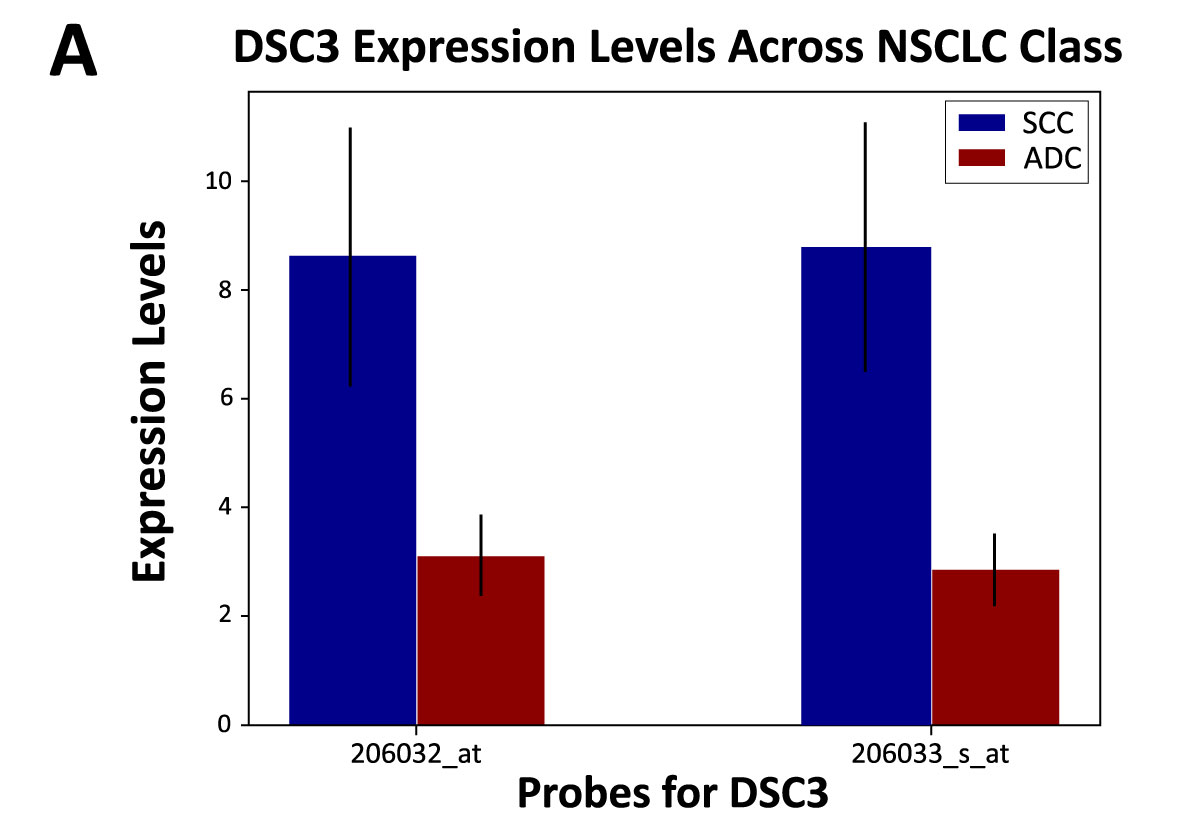
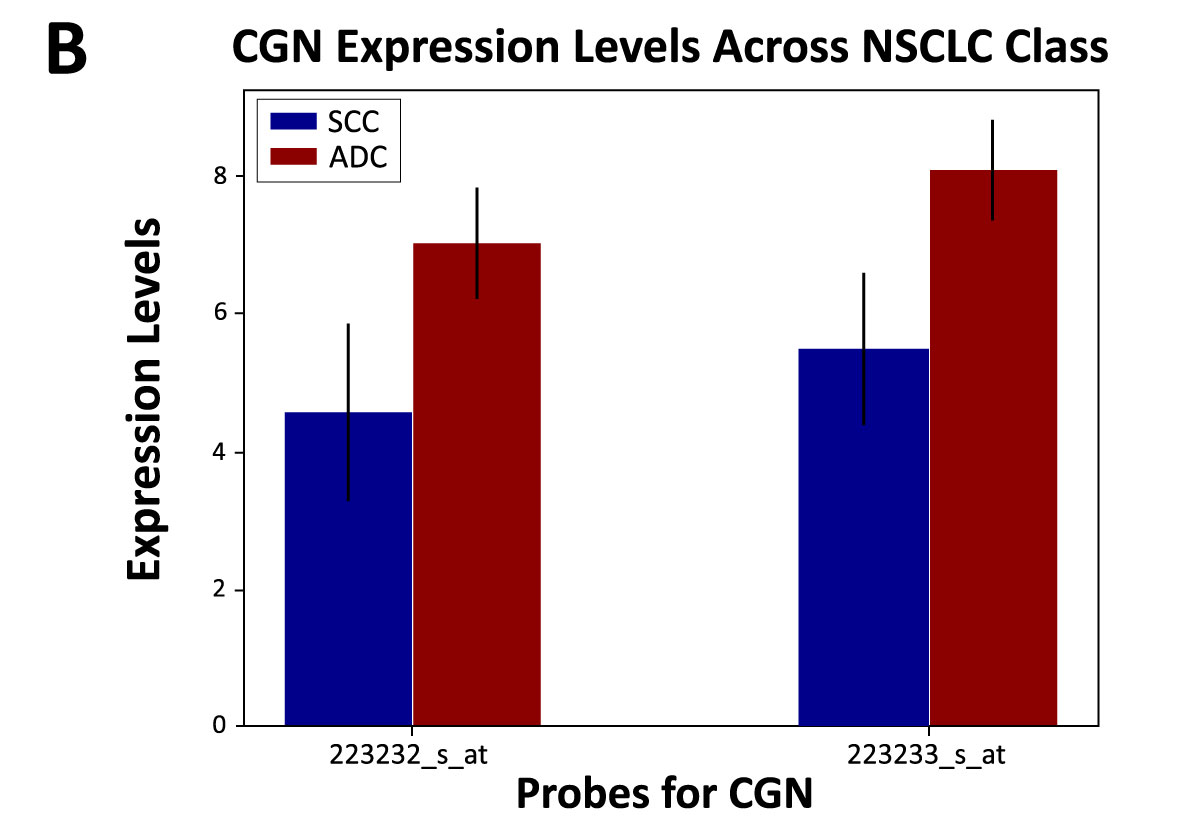
Figure 2. DSC3 and CGN expression in SCC and ADC patients.
Identification of PIGX as a Novel Genetic Driver Associated with NSCLC
One of the noteworthy findings in this study was PIGX, a gene that has not been previously associated with NSCLC. However, PIGX has been reported to promote cancer cell proliferation by suppressing EHD2 and ZIC1 in breast cancer.8 Our analyses found that PIGX was able to distinguish between ADC and SCC by being overexpressed in SCC patients. This finding from a small patient dataset highlights a novel gene that warrants further investigation for the advancement of precision medicine in NSCLC.
Significance of Using NetraAI Generate Hypotheses for NSCLC
The NetraAI’s ability to generate hypotheses about patient populations from small datasets allows researchers to explore the valuable information that lies within them that are otherwise skipped with traditional ML methodologies. Adopting this approach can assist in extracting meaningful insights quicker, including replicating genetic drivers of the ADC and SCC subtypes, identifying novel genetic drivers of these subgroups, highlighting sex-based differences, as well as uncovering novel genetic drivers, all of which can help us move closer toward precision medicine.
References
-
- Ridge, C., McErlean, A. M. & Ginsberg, M. S. Epidemiology of lung cancer. Semin Intervent Radiol 30, 93–98 (2013).
- Moses, C. et al. Small Patient Datasets Reveal Genetic Drivers of Non-Small Cell Lung Cancer Subtypes Using Machine Learning for Hypothesis Generation. Explor Med (2023).
- Hu, F. et al. Gene Expression Classification of Lung Adenocarcinoma into Molecular Subtypes. IEEE/ACM Trans Comput Biol Bioinform 17, 1187–1197 (2020).
- Kuner, R. et al. Global gene expression analysis reveals specific patterns of cell junctions in non-small cell lung cancer subtypes. Lung Cancer 63, 32–38 (2009).
- Sanchez-Palencia, A. et al. Gene expression profiling reveals novel biomarkers in nonsmall cell lung cancer. Int J Cancer 129, 355–364 (2011).
- Liu, Y. et al. Interferon regulatory factor 6 correlates with the progression of non-small cell lung cancer and can be regulated by miR-320. J Pharm Pharmacol 73, 682–691 (2021).
- Kuner, R. et al. Global gene expression analysis reveals specific patterns of cell junctions in non-small cell lung cancer subtypes. Lung Cancer 63, 32–38 (2009).
- Nakakido, M. et al. Phosphatidylinositol glycan anchor biosynthesis, class X containing complex promotes cancer cell proliferation through suppression of EHD2 and ZIC1, putative tumor suppressors. Int J Oncol 49, 868–876 (2016)